Generative designs of diverse materials
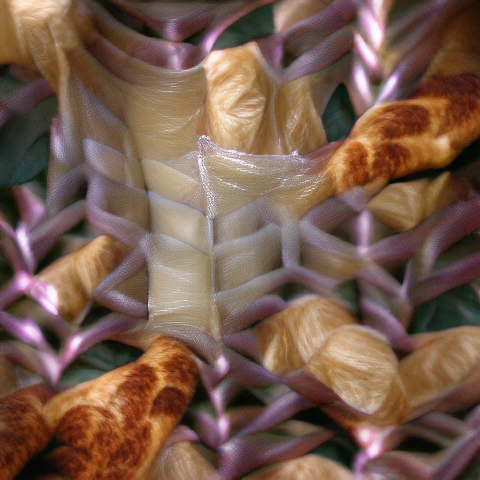
In the paper “Front. Mater., 2021”, we demonstrate the use of transformer neural networks in the de novo design of architected materials using a unique approach based on text input that enables the design to be directed by descriptive text, such as “a regular lattice of steel”. Since transformer neural nets enable the conversion of data from distinct forms into one another, including text into images, such methods have the potential to be used as a natural-language-driven tool to develop complex materials designs. In this study we use the Contrastive Language-Image Pre-Training (CLIP) and VQGAN neural networks in an iterative process to generate images that reflect text prompt driven materials designs. We then use the resulting images to generate three-dimensional models that can be realized using additive manufacturing, resulting in physical samples of these text-based materials. We present several such word-to-matter examples, and analyze 3D printed material specimen through associated additional finite element analysis, especially focused on mechanical properties including mechanism design. As an emerging new field, such language-based design approaches can have profound impact, including the use of transformer neural nets to generate machine code for 3D printing, optimization of processing conditions, and other end-to-end design environments that intersect directly with human language.
In the paper “APL. Mater., 2022”, we describe a method to generate 3D architected materials based on mathematically parameterized human readable word input, offering a direct materialization of language. Our method uses a combination of a vector quantized generative adversarial network and contrastive language-image pre-training neural networks to generate images, which are translated into 3D architectures that are then 3D printed using fused deposition modeling into materials with varying rigidity. The novel materials are further analyzed in a metallic realization as an aluminum-based nano-architecture, using molecular dynamics modeling and thereby providing mechanistic insights into the physical behavior of the material under extreme compressive loading. This work offers a novel way to design, understand, and manufacture 3D architected materials designed from mathematically parameterized language input. Our work features, at its core, a generally applicable algorithm that transforms any 2D image data into hierarchical fully tileable, periodic architected materials. This method can have broader applications beyond language-based materials design and can render other avenues for the analysis and manufacturing of architected materials, including microstructure gradients through parametric modeling. As an emerging field, language-based design approaches can have a profound impact on end-to-end design environments and drive a new understanding of physical phenomena that intersect directly with human language and creativity. It may also be used to exploit information mined from diverse and complex databases and data sources
In our recent paper which is still under review, we further extend the applications of transformer-based model to generate de novo protein designs using voxel-based representation. We report a method to generate de novo protein designs through a generative adversarial neural network, MolShapeGAN, that can rapidly produce a large variety of nanoarchitected material designs inspired by proteins. The proteomic molecular designs generated by MolShapeGAN model are examined using LAMMPS coarse-grained simulations by applying tensile deformation to the longest axis of each structure, to assess mechanical properties. In order to facilitate nanomechanical optimization, we develop a transformer neural network, denoted as VoxelPerceiver, that predicts mechanical properties directly from the molecular architecture in an end-to-end fashion. The assessment of key nanomechanical properties, such as maximum tensile stress, Von Mises stress mean, and Von Mises standard deviation, offer a materiomic design paradigm by which tailored nanomechanical properties can be achieved, and by which important insights can be gained about the particularities of nanomechanical responses of molecular structures. Optimization to achieve desired mechanical properties is performed both using a brute-force grid search and Bayesian optimization. We also report manufactured samples of scaled-up architected models of the protein designs using 3D printing.